Solutions for every business need
Growth comes in all shapes and sizes. Whether it’s smoother operations, sharper insights, higher revenue—or all of the above—we’ve got you covered

Warehouse management made simple
Gain full control over your inventory with smart automation, real-time tracking, and efficient workflows—ensuring your stock moves smoothly, every time

Launch hyperlocal commerce instantly
Set up and scale your hyper-local e-commerce with easy tools for web, orders, and delivery management—bringing you closer to customers, faster

Delivery management made easy
Gain control over your deliveries with real-time visibility, smart automation, and seamless coordination—ensuring on-time arrivals, every time
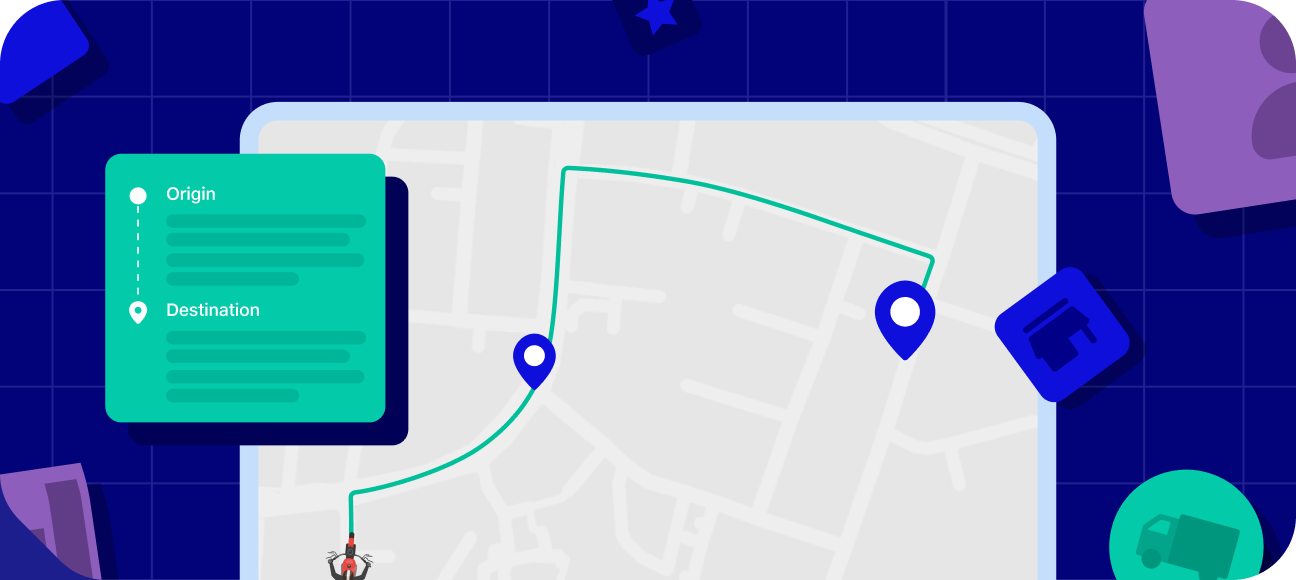
Order management made easy
Master every order with full visibility, smart automation, and effortless control—delivering the right outcomes, every time
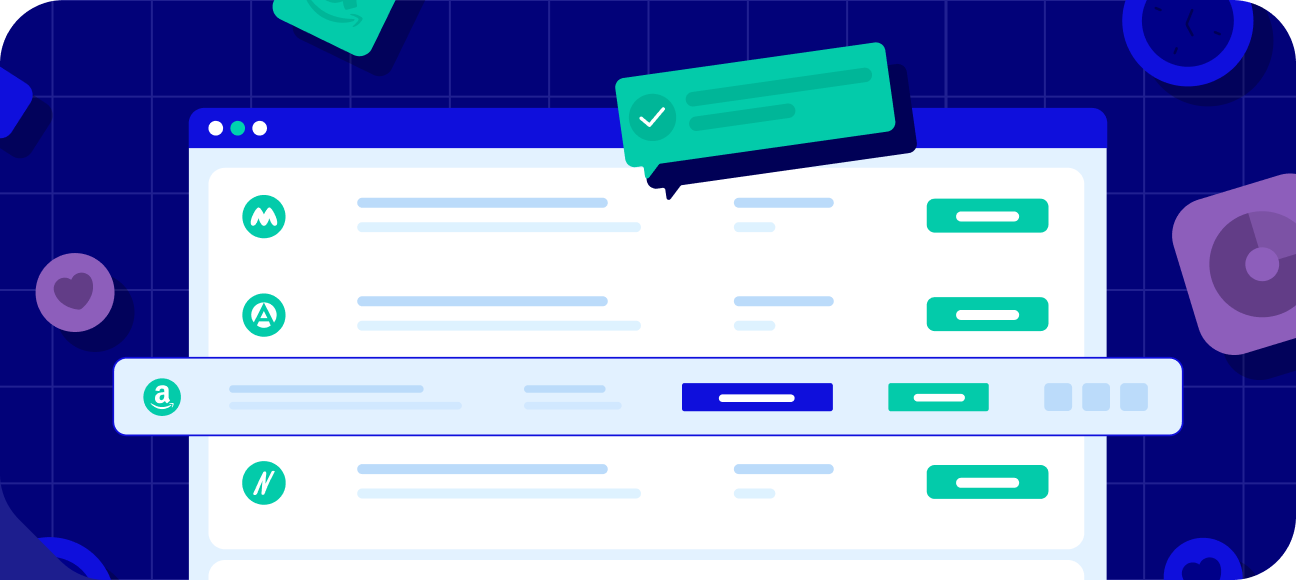
Speak to an expert
Explore how Fynd Commerce can improve every aspect of your business, from online to instore and beyond
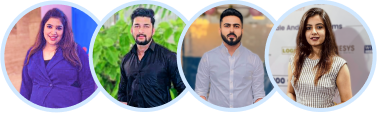
Speak to an expert
Explore how Fynd WMS powers operational excellence with our solution experts
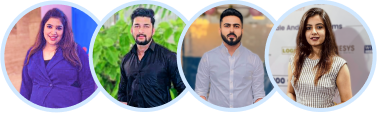
Speak to an expert
Explore how Fynd Quick powers hyperlocal businesses with our solution experts
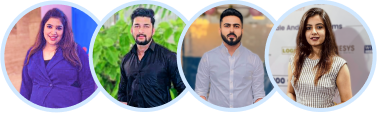
Speak to an expert
Explore how Fynd TMS powers better deliveries with our solution experts
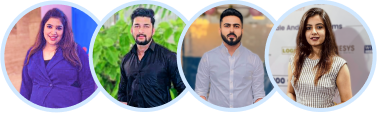
Speak to an expert
Explore how Fynd OMS powers seamless order management with our solution experts
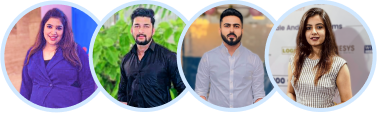
Featured Blog

Empower your business, every step of the way
Simplify operations, scale smarter, and deliver better experiences with solutions designed to grow with your business
Take your warehouse management to the next level
Experience hassle-free inventory management with Fynd WMS, designed to keep your operations efficient and accurate. Book a demo to see how Fynd WMS can boost productivity and profits for your business!
Kickstart your quick delivery website
Experience seamless hyper-local commerce with Fynd Quick—engineered to keep things running smoothly and help you reach customers in record time. Book a demo and see how Fynd Quick can drive efficiency and growth for your business!
Level up your delivery management
See how you can manage deliveries with Fynd TMS, designed to streamline operations and keep your customers delighted. Book a demo with our team and find out how Fynd TMS can boost your efficiency and bottom line!
Take your order management to the next level
Discover how effortless order management can be with Fynd OMS, built to keep things running smoothly and make your customers happy. Book a demo with our team and see how Fynd OMS can help boost your efficiency and profits!
A data warehouse is a specialized data management system designed to support business intelligence (BI) and analytics. Built to handle large-scale queries and analyses, it typically stores vast amounts of historical data. This data usually comes from a variety of sources, including application logs, transactional applications, and other databases, creating a unified repository for business insights.
By centralizing data from multiple sources, data warehouses streamline data management and provide a powerful foundation for analytics. Organizations can leverage this consolidated data to uncover patterns, track performance metrics, and enhance decision-making processes. The analytical tools within a data warehouse are especially useful for teams looking to make informed, data-driven decisions.
Over time, a data warehouse builds an extensive historical record, making it a reliable “single source of truth” for the organization. This historical archive is invaluable to data scientists and business analysts, enabling them to track trends, model projections, and refine business strategies based on comprehensive and consistent data.
Data Warehouse Defined
A data warehouse is a centralized repository that stores vast amounts of structured and unstructured data from multiple sources within an organization. It is designed to facilitate reporting and data analysis, allowing users to access and analyze data efficiently. Unlike traditional databases optimized for transactional processes, data warehouses are structured to support complex queries and business intelligence activities, enabling organizations to make informed decisions based on comprehensive data insights.
The architecture of a data warehouse typically includes components such as data integration, ETL (extract, transform, load) processes, and data marts, which are subsets of data warehouses tailored for specific business needs. By consolidating data from various sources into a unified system, a data warehouse enhances data consistency, improves data quality, and allows for historical data analysis, making it a critical tool for organizations aiming to leverage data for strategic advantage.
Benefits of a Data Warehouse
Data warehouses offer numerous benefits that significantly enhance an organization’s ability to manage and analyze data effectively. By centralizing data from various sources, they provide users with accurate and consistent information, which is crucial for informed decision-making.
This integration improves data quality and supports advanced analytics and reporting capabilities, enabling businesses to identify trends and make strategic choices. Additionally, data warehouses streamline operations by providing a unified view of data, allowing for more efficient data retrieval. Ultimately, these advantages position organizations to thrive in today’s competitive, data-driven landscape, leveraging insights for better performance and growth.
Enhanced Data Quality
Data warehouses significantly improve data quality by employing rigorous ETL processes that clean, transform, and integrate data from various sources. This ensures that information is accurate, consistent, and reliable, reducing the chances of errors in analysis. With higher data quality, organizations can trust their insights, leading to better-informed decision-making and strategic planning that positively impacts overall business performance.
Improved Decision-Making
A centralized data warehouse provides a comprehensive view of organizational data, enabling users to make informed decisions quickly. By accessing integrated information from multiple sources, decision-makers can identify trends, patterns, and insights that might otherwise be overlooked. This enhanced visibility fosters data-driven decision-making, allowing businesses to respond proactively to market changes and align strategies with organizational goals.
Historical Analysis
Data warehouses facilitate historical analysis by storing vast amounts of historical data over time. This capability allows organizations to analyze past trends, performance metrics, and customer behavior, providing valuable insights for forecasting future outcomes. By understanding historical patterns, businesses can make more accurate predictions, refine their strategies, and better allocate resources to capitalize on emerging opportunities.
Faster Query Performance
Optimized for complex queries, data warehouses deliver superior query performance compared to traditional databases. This efficiency is crucial for businesses that require timely access to data for reporting and analytics. Faster query response times enable users to retrieve insights quickly, streamlining decision-making processes and enhancing overall productivity. As a result, organizations can respond to changing business needs with agility and precision.
Scalability
Data warehouses are designed to be scalable, accommodating growing data volumes as organizations expand. This scalability ensures that businesses can manage increasing data demands without compromising performance or efficiency. By easily integrating new data sources and supporting larger datasets, data warehouses enable organizations to adapt to evolving market conditions and harness the power of big data, ensuring long-term viability and success.
Where are Data Warehouses Stored?
Data warehouses can be stored in various environments, primarily categorized as on-premises, cloud-based, or hybrid solutions. In on-premises setups, organizations host data warehouses within their own data centers, using dedicated hardware and infrastructure. This approach allows for greater control over data security, compliance, and customization but often requires significant upfront investment and ongoing maintenance.
Alternatively, cloud-based data warehouses are hosted by third-party providers, offering flexibility, scalability, and reduced infrastructure costs. Organizations can access their data warehouses over the Internet, benefiting from the provider's resources and expertise in data management.
What are the Key Differences Between OLTP and Data Warehouse (OLAP) Systems?
Online Transaction Processing (OLTP) systems and Online Analytical Processing (OLAP) systems serve distinct functions in the realm of data management and processing. OLTP systems are designed for managing and facilitating real-time transactional operations, such as order processing, inventory management, and customer transactions.
In contrast, data warehouses, or OLAP systems, are designed to support complex queries and analytical operations, making them ideal for decision support and business intelligence activities. OLAP systems store large volumes of historical data that are often aggregated from various sources, enabling organizations to analyze trends, patterns, and relationships over time.
Traditional vs. Cloud-based Data Warehouse
Traditional data warehouses are on-premises solutions that require significant hardware investments and ongoing maintenance. These systems often involve complex setup processes and lengthy deployment times. While they provide organizations with control over their data and infrastructure, they can be costly to scale and may lack the flexibility needed to adapt to changing business needs. Additionally, organizations are responsible for managing backups, security, and updates, which can strain IT resources.
In contrast, cloud-based data warehouses offer a more flexible and cost-effective solution. These services provide on-demand scalability, allowing organizations to adjust their storage and computing resources based on current needs. Cloud-based systems also simplify management by automating maintenance tasks such as backups and security updates. Furthermore, they enable easier access to advanced analytics tools and integration with other cloud services, fostering collaboration and innovation. Overall, while traditional data warehouses provide control, cloud-based solutions deliver enhanced flexibility, scalability, and efficiency.
What Does a Typical Data Warehouse Implementation Look Like
A typical data warehouse implementation is a structured process designed to create a centralized repository for an organization’s data, enabling efficient data analysis and reporting. The implementation journey begins with a thorough understanding of business requirements and the identification of relevant data sources.
This involves careful planning, data modeling, and designing the architecture to ensure that the data warehouse meets the analytical needs of the organization. Following this, the extraction, transformation, and loading (ETL) of data take place, culminating in a robust data warehouse that supports informed decision-making and drives business intelligence initiatives.
1. Requirements Gathering
The implementation process begins with gathering requirements from stakeholders to understand their data needs and business objectives. This phase involves interviewing users, analyzing existing data sources, and identifying key performance indicators (KPIs) that the data warehouse will support. By comprehensively documenting these requirements, the organization ensures that the data warehouse aligns with business goals, facilitating effective decision-making and reporting.
2. Data Modeling
Once requirements are established, the next step is to design the data model for the warehouse. This involves creating a logical and physical representation of the data, including defining dimensions, facts, and relationships. Data modeling ensures that the structure of the warehouse supports efficient data retrieval and analysis. It often includes selecting an appropriate schema, such as a star or snowflake, to optimize query performance and enhance user experience.
3. ETL Process
The Extract, Transform, Load (ETL) process is a crucial phase in data warehouse implementation. During this stage, data is extracted from various sources, including databases, flat files, and external systems. The extracted data undergoes transformation, where it is cleaned, validated, and formatted to ensure consistency and accuracy. Finally, the transformed data is loaded into the data warehouse organized according to the predefined schema, making it readily available for analysis and reporting.
4. Data Warehouse Design
Data warehouse design involves creating a framework for how data will be stored, accessed, and organized. This includes determining the architecture, such as star or snowflake schemas, which influences query performance and data retrieval efficiency. Design considerations also encompass indexing, partitioning, and data distribution strategies to optimize performance. A well-designed data warehouse accommodates future growth and provides a user-friendly environment for data analysts and business users.
5. Testing and Validation
After the data warehouse is built, rigorous testing and validation are essential to ensure that it meets business requirements and functions correctly. This phase includes verifying data accuracy, consistency, and completeness through various tests, such as unit testing, system testing, and user acceptance testing (UAT). Addressing any discrepancies or performance issues during this stage helps prevent potential problems in production and ensures that stakeholders can rely on the data warehouse for decision-making.
6. Deployment
Once testing is complete and any issues have been resolved, the data warehouse is deployed for use by the organization. This phase involves configuring user access, setting up security protocols, and integrating the data warehouse with existing systems and applications. Training users on how to navigate and utilize the data warehouse effectively is also crucial during deployment. A successful deployment ensures that the data warehouse is operational and accessible for ongoing analysis and reporting.
7. Maintenance and Support
Post-deployment, ongoing maintenance, and support are vital for ensuring the data warehouse continues to perform optimally. This includes monitoring performance, managing data storage, and addressing any emerging issues or user feedback. Regular updates and enhancements may be necessary to accommodate new data sources or changing business requirements. By maintaining the data warehouse effectively, organizations can maximize its value and ensure it remains a critical resource for data-driven decision-making.
Schemas in Data Warehouses
Schemas in data warehouses are essential frameworks that define how data is organized, stored, and accessed. They provide a structured blueprint for arranging information into tables, fields, and relationships, facilitating efficient data retrieval and analysis. By utilizing various schema designs, organizations can optimize performance and improve the user experience when querying data.
The most common schema types include star schema, snowflake schema, and galaxy schema, each offering unique advantages based on specific analytical needs and performance requirements. Understanding these schemas is crucial for designing a data warehouse that effectively supports business intelligence and decision-making processes.
Star Schema
The star schema is one of the simplest and most widely used designs in data warehousing. It consists of a central fact table that holds quantitative data, surrounded by dimension tables that contain descriptive attributes related to the facts. This design simplifies queries and enhances performance, as it allows for straightforward joins between the fact and dimension tables, making it easy for users to perform analyses and generate reports.
Snowflake Schema
The snowflake schema is a more normalized version of the star schema, where dimension tables are further broken down into related sub-dimensions. This structure reduces data redundancy and improves data integrity by organizing information into additional tables. While the snowflake schema can enhance data organization and storage efficiency, it may lead to more complex queries and slower performance due to the multiple joins required when accessing data.
Galaxy Schema
The galaxy schema, also known as a fact constellation schema, combines multiple star schemas into a single structure. This approach allows for the modeling of complex relationships and diverse business processes within a single data warehouse. By having multiple fact tables linked to various dimension tables, the galaxy schema provides a flexible framework for comprehensive data analysis. It is particularly beneficial for organizations with extensive and varied analytical requirements.
Fact and Dimension Tables
Fact tables and dimension tables are essential components of data warehouse schemas. Fact tables store quantitative data, such as sales revenue or transaction counts, and often include foreign keys linking to related dimension tables. Dimension tables, on the other hand, contain descriptive attributes that provide context for the facts, such as customer names, product details, or periods. Together, these tables enable effective data analysis and reporting.
Choosing the Right Schema
Selecting the appropriate schema for a data warehouse depends on various factors, including the organization's analytical requirements, data volume, and performance needs. A star schema may be ideal for straightforward reporting and fast query performance, while a snowflake schema can enhance data organization and storage efficiency. Understanding the strengths and weaknesses of each schema type allows organizations to design a data warehouse that best supports their data analysis and reporting objectives.
Components of Data Warehouse Architecture
Data warehouse architecture consists of several key components that work together to facilitate efficient data storage, processing, and analysis. At its core, the architecture includes data sources, ETL (Extract, Transform, Load) processes, the data warehouse itself, and data presentation layers.
Each component plays a critical role in ensuring that data is accurately gathered, transformed, and made accessible for analytical purposes. Additionally, there are data marts, which are specialized subsets of data warehouses tailored to specific business needs. A well-designed data warehouse architecture enables organizations to consolidate their data, support business intelligence initiatives, and drive informed decision-making.
1. Data Sources
Data sources are the foundational elements of data warehouse architecture, providing the raw data necessary for analysis. These sources can include operational databases, external data feeds, flat files, and online services. By integrating data from multiple sources, organizations can create a comprehensive view of their operations, ensuring that the data warehouse is populated with accurate and relevant information for decision-making and reporting.
2. ETL Processes
ETL (Extract, Transform, Load) processes are critical for preparing data for the data warehouse. During the extraction phase, data is gathered from various sources and consolidated into a staging area. In the transformation phase, the data is cleaned, validated, and formatted to ensure consistency and accuracy. Finally, in the loading phase, the transformed data is loaded into the data warehouse, making it ready for analysis and reporting.
3. Data Warehouse
The data warehouse is the central repository that stores integrated data from multiple sources, organized in a way that supports efficient querying and analysis. It typically employs a denormalized structure, allowing for faster access to data for reporting purposes. The data warehouse is designed to handle large volumes of historical data, enabling organizations to perform complex analyses and generate valuable insights for decision-making.
4. Data Marts
Data marts are specialized subsets of the data warehouse tailored to specific business functions or departments, such as sales, marketing, or finance. They allow users to access relevant data quickly without sifting through the entire data warehouse. Data marts can enhance performance by streamlining data retrieval and providing focused analytical capabilities. They can be created from the main data warehouse or independently, depending on organizational needs.
5. Presentation Layer
The presentation layer is the user interface that allows business users to access, analyze, and visualize data stored in the data warehouse. This layer includes reporting tools, dashboards, and data visualization applications that facilitate data exploration and insights. A well-designed presentation layer enables users to generate reports, conduct ad-hoc analyses, and share findings with stakeholders, driving informed decision-making and fostering a data-driven culture within the organization.
Use Cases For Data Warehouses
Data warehouses are versatile tools that support a wide range of business use cases, enabling organizations to make data-driven decisions and optimize operations. They serve as centralized repositories for integrating data from multiple sources, allowing for complex analysis and reporting.
Common use cases include business intelligence and reporting, data mining, customer analytics, financial analysis, and trend analysis. By leveraging data warehouses, organizations can uncover valuable insights, identify opportunities, and enhance overall performance.
Business Intelligence and Reporting
Data warehouses serve as the backbone for business intelligence (BI) initiatives, providing a centralized location for data analysis and reporting. Organizations can generate comprehensive reports and dashboards that offer insights into key performance indicators (KPIs) and operational metrics. By consolidating data from various sources, data warehouses enable stakeholders to make informed decisions based on accurate and up-to-date information.
Data Mining
Data warehouses facilitate data mining by providing a rich repository of historical and current data that can be analyzed to discover patterns, trends, and correlations. Analysts can use advanced algorithms and statistical methods to identify insights that inform strategic decisions, such as customer segmentation, market trends, and product performance. This capability enhances organizations' ability to leverage data for competitive advantage.
Customer Analytics
Data warehouses enable organizations to conduct in-depth customer analytics by consolidating data from multiple touchpoints, including transactions, interactions, and demographics. This comprehensive view allows businesses to understand customer behavior, preferences, and purchasing patterns. With these insights, organizations can tailor marketing strategies, improve customer experiences, and enhance customer retention efforts, ultimately driving revenue growth.
Financial Analysis
Financial analysis is another critical use case for data warehouses, as they provide a centralized repository for financial data, including budgets, forecasts, and historical performance. Organizations can analyze financial trends, conduct variance analysis, and generate reports to support strategic planning and decision-making. By leveraging a data warehouse, finance teams can ensure data accuracy, improve compliance, and streamline reporting processes.
Trend Analysis
Data warehouses are ideal for conducting trend analysis, allowing organizations to analyze historical data over time and identify patterns or shifts in performance. This capability enables businesses to forecast future outcomes, assess the impact of external factors, and make proactive decisions based on data-driven insights. By leveraging trend analysis, organizations can enhance their strategic planning and adapt to changing market conditions effectively.
Conclusion
Data warehouses are essential for organizations looking to harness the power of their data for informed decision-making and improved operational efficiency. By centralizing data from various sources and supporting advanced analytics, data warehouses empower businesses to uncover insights, identify trends, and drive strategic initiatives.
As organizations continue to prioritize data-driven strategies, the role of data warehouses will remain vital in enhancing business intelligence and enabling competitive advantage in today's dynamic marketplace.
Frequently asked questions
A data warehouse is a centralized repository that stores integrated data from multiple sources, enabling efficient querying, reporting, and analysis for informed decision-making and business intelligence.
A data warehouse is designed for analytical processing and reporting, while a database focuses on transaction processing. Data warehouses store historical data, whereas databases manage current operational data.
Key components include data sources, ETL processes, the data warehouse itself, data marts, and the presentation layer, each playing a crucial role in data storage and analysis.
ETL stands for Extract, Transform, Load. It is the process of extracting data from various sources, transforming it into a suitable format, and loading it into the data warehouse.
Common use cases include business intelligence and reporting, data mining, customer analytics, financial analysis, and trend analysis, all aimed at improving decision-making and operational efficiency.
A data warehouse centralizes data from multiple sources, providing accurate and up-to-date information for analysis. This enables organizations to generate reports and insights that inform strategic decisions.